Research
The WISELab work on a broad range of theoretical problems in signal, optimization, and machine learning for wireless communications and localization. The focus is on two main research pillars: (i) radio map learning and tomography, and (ii) dynamic 3D communications, with target industrial applications in 6G and beyond.
Radio map learning and application
How to enhance the intelligence for wireless communication networks? One promising direction is to fuse more environmental information to the network, such as constructing geography-aware radio maps. Radio map is a data model that describes the wireless communication quality between any transmitter and receiver location pair. The technology has been successfully applied for network planning, spectrum management, and fingerprint localization for over 20 years. However, conventional radio map techniques were limited to the case where the transmitter locations are fixed and the maps are usually 2-D for varying the receiver location on the ground. Such a radio map is blind to the actual geographic environment, limiting the choice of control actions for adapting the network to the environment. Besides, prevalent methods are heavily model dependent, limiting its robustness in uncertain environments. Our goal is to construct simultaneously a radio map and an virtual environment map based on sensing from radio signals, where the virtual environment map represents objects “seen” by radio signals via radio tomography. Theoretically, our lab endeavor to advance the knowledge in the following directions:
Modeling and optimization for radio map estimation
Deep learning for radio map construction
Applications in communications and localization
Selected recent work
Tomographic imaging from UAV assisted RF sensing
P. Zeng and J. Chen, “UAV-aided Joint Radio Map and 3D Environment Reconstruction using Deep Learning Approaches”, to appear in Proceedings of IEEE International Conference on Communications, 2022.
In this paper, a model-driven deep learning (DL) model is developed for joint radio map learning and 3D environment reconstruction. The challenge mainly comes from the high dimensionality of the radio map for characterizing radio links between transmitters and receivers with full spatial degrees of freedom. A framework with model-assisted deep neural network (DNN) structures to learn and memorize the 3D virtual obstacle environment is proposed. A semi-self-adaptive training strategy is developed for the proposed DL-based radio map, which demonstrates robustness over existing model-based radio map construction methods. Numerical results show that our DL framework outperforms pure model-based method in terms of higher radio map reconstruction accuracy and computation efficiency.
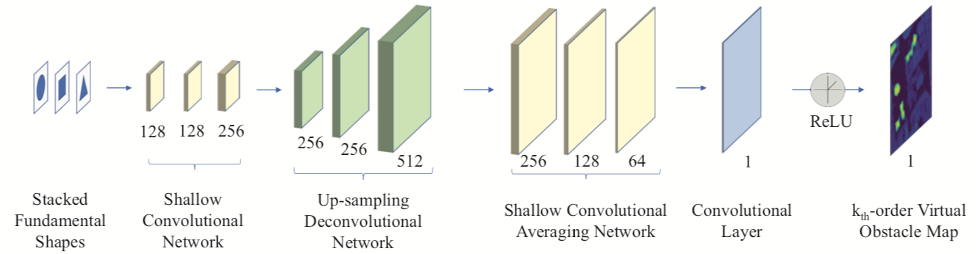
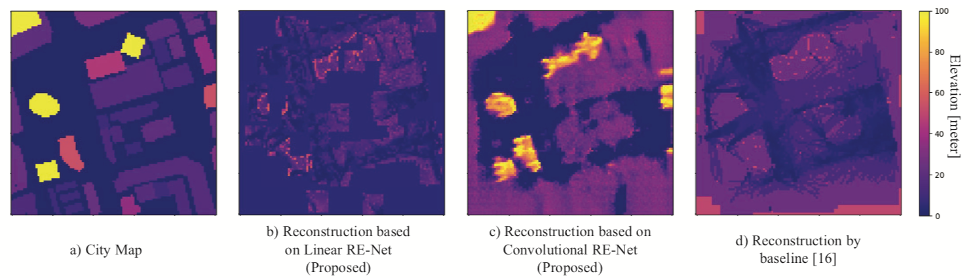
Propagation field reconstruction via matrix completion
H. Sun and J. Chen, “Regression assisted matrix completion for reconstructing a propagation field with application to source localization” to appear in Proceeding of IEEE International Conference on Acoustic, Speech, and Signal Processing, 2022.
This paper develops a regression assisted matrix completion method to reconstruct the propagation field for received signal strength (RSS) based source localization without prior knowledge of the propagation model. Existing matrix completion methods did not exploit the fact that the uncertainty of each observed entry is different due to the reality that the sensor density may vary across different locations. This paper pro- poses to employ local polynomial regression to increase the accuracy of matrix completion. First, the values of selected entries of a matrix are estimated via interpolation from local measurements, and the interpolation error is analyzed. Then, a matrix completion problem that is aware of the different uncertainty of observed entries is formulated and solved.
|
It is demonstrated that the proposed method significantly improves the performance of matrix completion, and thus ultimately achieving a better reconstruction for the propagation field. In application to underwater localization based on RSS measurements from sensor networks, the proposed scheme demonstrates 2X accuracy than baselines.
|
Dynamic 3D Communications
The recent success of commercial UAV applications motivates a new dimension of optimizing wireless networks, where the network dynamically optimizes the node positions for enhanced communication capability. This can be done by introducing a flying relay to connect two geographically separated ground users. The fundamental challenge is to avoid propagation blockage due to various objects in dense urban area, where blockage will be a vital issue under the deployment of millimeter-wave, terahertz, and free-space optical air interfaces.
Future communication networks, with the additional spatial degree-of-freedom offered by the UAV radio nodes, shall adapt its topology to the geometry of the environment. However, the locations and shapes of the environment objects can be arbitrary and very complicated. Our lab strives to tackle the following challenges:
Geography-aware air-to-ground channel modeling, estimation, and adaptation
UAV placement and trajectory optimization
Integration with massive MIMO, intelligent reflective surface, and so on.
Solutions to these problems require synergies between analytic geometry, communication theory, machine learning, and optimizations. Check out our recent papers for some preliminary results:
B. Zhang and J. Chen, “Constructing Radio Maps for UAV Communications via Dynamic Resolution Virtual Obstacle Maps”, in Proc. SPAWC, 2020.
J. Chen and D. Gesbert, ” Efficient Local Map Search Algorithms for the Placement of Flying Relays”, IEEE Trans. Wireless Commun., vol. 19, no. 2, 2020. [Preprint][Slides][IEEE]
J. Chen, U. Mitra, and D. Gesbert, “3D Urban UAV Relay Placement: Linear Complexity Algorithm and Analysis”, IEEE Trans. Wireless Commun., vol. 20, no. 8, 2021. [IEEE]
More preliminary/related results
Deep learning for prediction in 5G massive MIMO networks
This work predicts the spectrum efficiency (SE) for 5G new radio (NR) cellular networks based on the channel state information (CSI) statistics, such as reference signal received power (RSRP) and average channel quality information (CQI), are studied. The problem is challenging because there is no clear correspondence between the CSI statistics and the SE in a 5G NR network. Instead, there are many instantaneous factors that affect the SE, such as the adaptive beamforming strategy employed by the base station (BS) and the inter-cell interference due to the traffic at the neighboring cell. In this paper, a model- assisted data-driven approach based on deep neural networks (DNN) is developed. First, a mobility-aware approach is derived to assist the data preprocessing and feature engineering; second, an interference-aware DNN model is developed to jointly train the predictions on the interference with noise and the SE. In our experiments in a real world 5G NR network, the BSs are equipped with 64 dual-polarized antennas, and a commercial user device with 4 antennas travels along a fixed route to collect drive testing measurements over a period of several months. It is found that the model-assisted approach indeed provides additional prediction accuracy of 1–2% over a purely data-driven approach, and the overall prediction error can be brought down to 15%.
Check out our recent paper:
Z. Xing, H. Li, W. Liu, Z. Ren, J. Chen, J, Xu, C. Qin, “Spectrum Efficiency Prediction for Real-World 5G Networks Based on Drive Testing Data”, in Proc. IEEE WCNC, 2022.
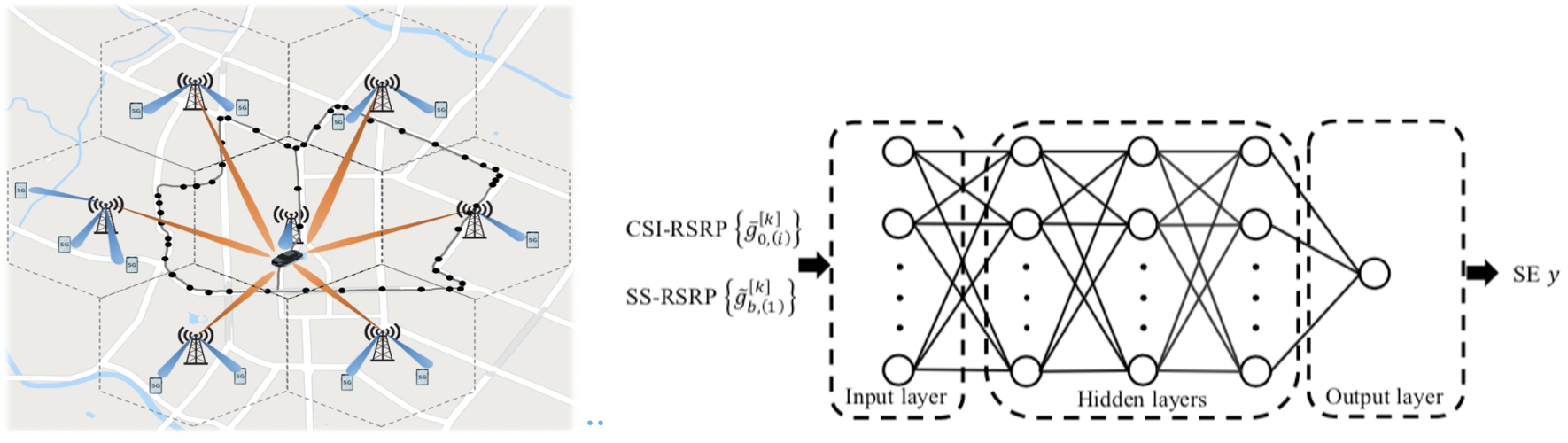
Non-parametric source localization from unimodal matrix completion
In practice for many applications, the signal propagation characteristics are not fully known due to the complex environment, such as for an acoustic signal in water. We proposed a model to arrange a number of measurements taken at random locations into a sparse matrix, and proved that the matrix has a unimodal property that can be leveraged to localize the source. We developed a non-parametric localization method based on a smart integration of various modern techniques: sparse matrix completion, eigenspace analysis, singular vector perturbation analysis, and peak localization. The method demonstrates robustness as well as order-wise reduction in the number of sensors over the state-of-the-art baselines.
|
We are now interested in fusing multimodal data, such as energy measurement and time-of-arrival measurement, for better localization performance. We are working on a tensor observation model and developing methods to mine a common unimodal structure using tensor decomposition techniques. Our preliminary result suggests that jointly processing the data in a tensor works better and separately manipulating each data matrix. See [J13, C15, C16, C18] [Slides] [Preprint].
|
Massive MIMO assisted by (device-to-device) D2D communications
It is a trend that user terminals have improving capability to communicate with each other directly via D2D communication protocols. While using D2D for data sharing may be too much a burden for the users, exploiting it to assist the signaling could be a trending solution. In particular, massive MIMO systems require a lot of signaling for the instantaneous channel state information (CSI) acquisition. We show that if the users can share a limited amount of CSI with each other via D2D, the downlink performance can be significantly improved in a limited feedback massive MIMO system under FDD mode. Specifically, we have solved three major problems: (i) For a limited amount of CSI exchange via D2D, whether it is better to feedback the quantized CSI or the precoder; (ii) How the users exchange the CSI efficiently, and (iii) How to design a good feedback and precoding strategy under practical D2D (finite rate and latency).
|
Preliminary results can be found in:
J. Chen, H. Yin, L. Cottatellucci, D. Gesbert, “Efficient feedback mechanisms for FDD massive MIMO under user-level cooperation,” IEEE Trans. Wireless Commun., 2017, [Preprint] [Code].
J. Chen, H. Yin, L. Cottatellucci, D. Gesbert, “Dual-regularized Feedback and Precoding for D2D Assisted MIMO Systems,” IEEE Trans. Wireless Commun. accepted, 2017, to appear. [Preprint] [Code]
J. Chen and V. K. Lau, “Two-tier precoding for FDD multi-cell massive MIMO time-varying interference networks,” IEEE J. Sel. Areas Commun., vol. 32, no. 6, pp. 1230–1238, Jun. 2014.
|
Cooperative Localization from A Game Perspective
Cooperation among users can significantly enhance the localization accuracy in GPS-challenging environments. The intuition is that the users can fuse the the location information by taking range measurements with each other. However, cooperation is resource consuming (power and bandwidth etc.) and the outcome is not necessarily fair among agent nodes. Our research addressed these issues by developing distributed link selection and power allocation strategies under different application scenarios. Specifically, we formulated serval resource management games according to different types of resource constraints in the network and studied their solution properties via analyzing game equilibria.
|
We found closed form conditions to determine whether a link should not be considered for cooperation; with that, a lot of coordination overheads among agents can be saved. In particular, we developed a novel link bargaining equilibrium concept, found sufficient conditions where the equilibrium is unique, and proposed iteration that can converge to the unique equilibrium. Such a novel game theoretic design and analysis framework may apply to other cooperation networks as well. [J9, J10, C8].
|
|